In today's digital age, the term "did buy" has become increasingly relevant in the world of e-commerce and consumer behavior analysis. The concept revolves around understanding what consumers have purchased, why they made the decision, and how businesses can leverage this information to improve their marketing strategies. This article delves deep into the "did buy" phenomenon, exploring its significance and providing actionable insights for businesses and consumers alike.
The phrase "did buy" is more than just a simple question; it represents a powerful tool for businesses to analyze consumer purchasing patterns. By understanding what customers have bought, companies can tailor their offerings, improve customer satisfaction, and ultimately drive revenue growth. This article will explore the various aspects of "did buy," including its implications for both businesses and consumers.
In this guide, we will examine the concept of "did buy" from multiple angles, including its definition, importance, and practical applications. Whether you're a business owner looking to optimize your sales strategies or a consumer curious about the factors influencing purchasing decisions, this article will provide valuable insights and actionable advice.
Read also:Will Cain Net Worth Exploring The Wealth And Career Of A Renowned Political Analyst
Understanding the "Did Buy" Concept
The term "did buy" refers to the act of analyzing what a consumer has purchased in the past. This concept is essential for businesses aiming to understand customer behavior and preferences. By examining past purchases, companies can gain insights into consumer needs, preferences, and trends, enabling them to make data-driven decisions.
Why Is "Did Buy" Important?
Understanding "did buy" is crucial for businesses because it allows them to:
- Predict future purchasing behavior.
- Optimize inventory management.
- Create targeted marketing campaigns.
- Improve customer satisfaction and loyalty.
Factors Influencing "Did Buy" Decisions
Several factors influence why consumers decide to "did buy." These include:
1. Price Sensitivity
Price is often a critical factor in purchasing decisions. Consumers are more likely to "did buy" when they perceive value for money. Businesses can leverage this by offering competitive pricing and discounts.
2. Brand Loyalty
Consumers who are loyal to a brand are more likely to "did buy" from that brand repeatedly. Building trust and providing excellent customer service can enhance brand loyalty.
Data Analysis in "Did Buy" Strategies
Data analysis plays a pivotal role in understanding "did buy" patterns. Businesses use various tools and techniques to analyze consumer data and gain insights into purchasing behavior.
Read also:Foot Croft Feet Your Ultimate Guide To Understanding And Managing Foot Health
Types of Data Used in "Did Buy" Analysis
The following types of data are commonly used in "did buy" analysis:
- Purchase history
- Demographic information
- Behavioral data
- Feedback and reviews
The Role of Technology in "Did Buy" Analysis
Technology has revolutionized the way businesses analyze "did buy" data. Advanced analytics tools and artificial intelligence (AI) enable companies to process vast amounts of data quickly and accurately.
Benefits of Using Technology for "Did Buy" Analysis
Some of the benefits of using technology in "did buy" analysis include:
- Improved accuracy in predictions
- Real-time data processing
- Enhanced customer segmentation
Challenges in Implementing "Did Buy" Strategies
While "did buy" strategies offer numerous benefits, there are challenges associated with their implementation. These include:
1. Data Privacy Concerns
Consumers are increasingly concerned about their data privacy. Businesses must ensure that they comply with data protection regulations and gain consumer trust.
2. Integration of Data Sources
Integrating data from various sources can be a complex process. Businesses need robust systems to ensure seamless data integration.
Case Studies: Successful "Did Buy" Strategies
Several companies have successfully implemented "did buy" strategies to boost their sales and customer engagement. Here are a few examples:
1. Amazon
Amazon uses advanced algorithms to analyze "did buy" data and provide personalized product recommendations to its customers. This has significantly enhanced customer satisfaction and increased sales.
2. Netflix
Netflix leverages "did buy" data to recommend movies and TV shows based on users' viewing history. This personalized approach has contributed to the platform's massive success.
Best Practices for "Did Buy" Implementation
To successfully implement "did buy" strategies, businesses should follow these best practices:
1. Invest in Data Analytics
Investing in advanced data analytics tools can help businesses gain deeper insights into consumer behavior and preferences.
2. Focus on Customer Experience
Providing an exceptional customer experience is key to encouraging repeat purchases. Businesses should prioritize customer satisfaction in their "did buy" strategies.
Future Trends in "Did Buy" Analysis
The future of "did buy" analysis looks promising, with emerging trends such as:
1. Artificial Intelligence
AI will continue to play a significant role in analyzing "did buy" data, enabling businesses to make more accurate predictions and personalized recommendations.
2. Augmented Reality
Augmented reality (AR) is set to transform the way consumers interact with products, influencing their "did buy" decisions.
Conclusion
In conclusion, understanding the concept of "did buy" is essential for businesses aiming to thrive in the competitive e-commerce landscape. By analyzing past purchases, companies can gain valuable insights into consumer behavior and preferences, enabling them to make informed decisions and enhance customer satisfaction.
We encourage readers to share their thoughts and experiences in the comments section below. Additionally, feel free to explore other articles on our website for more insights into consumer behavior and marketing strategies.
Table of Contents
- Understanding the "Did Buy" Concept
- Factors Influencing "Did Buy" Decisions
- Data Analysis in "Did Buy" Strategies
- The Role of Technology in "Did Buy" Analysis
- Challenges in Implementing "Did Buy" Strategies
- Case Studies: Successful "Did Buy" Strategies
- Best Practices for "Did Buy" Implementation
- Future Trends in "Did Buy" Analysis
- Conclusion
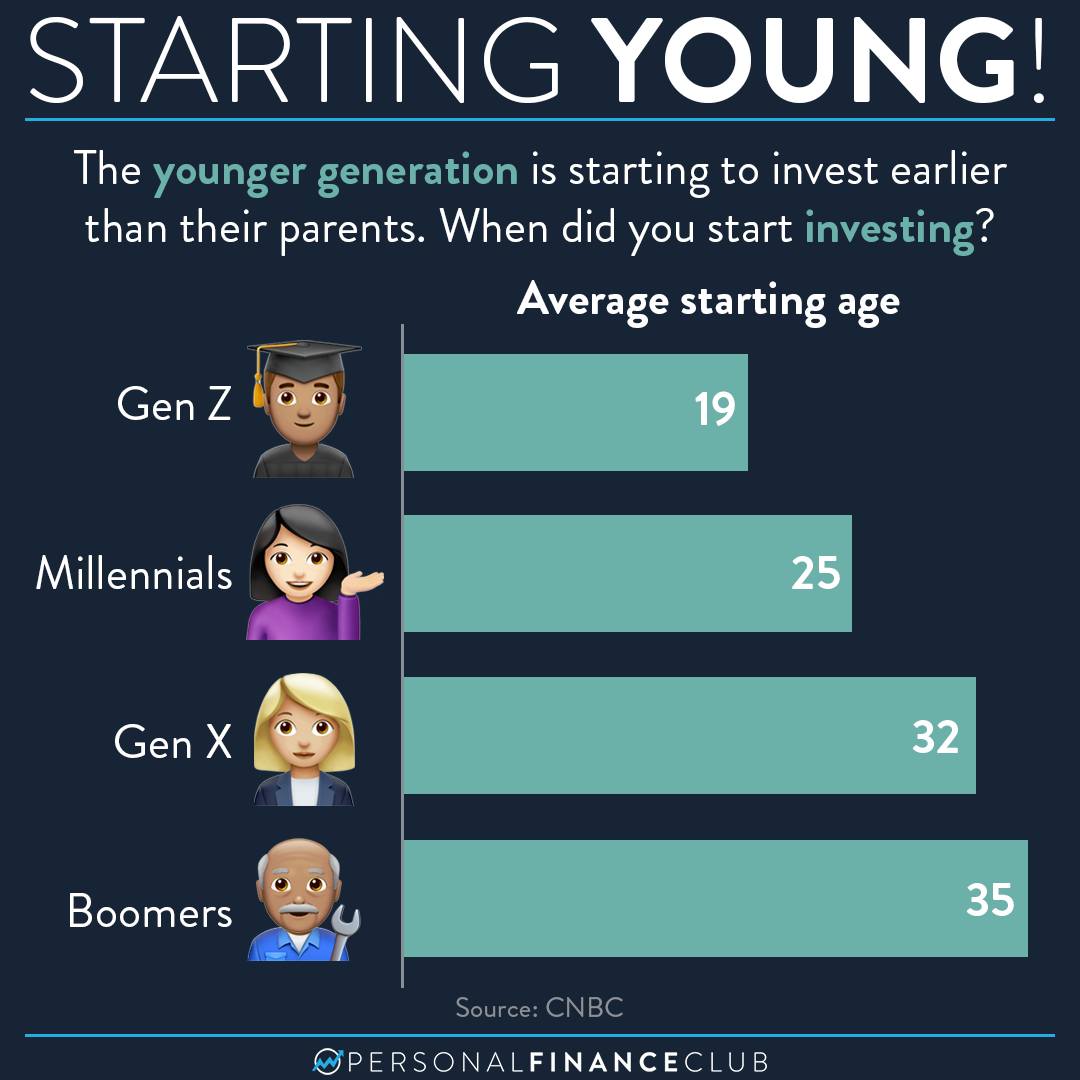
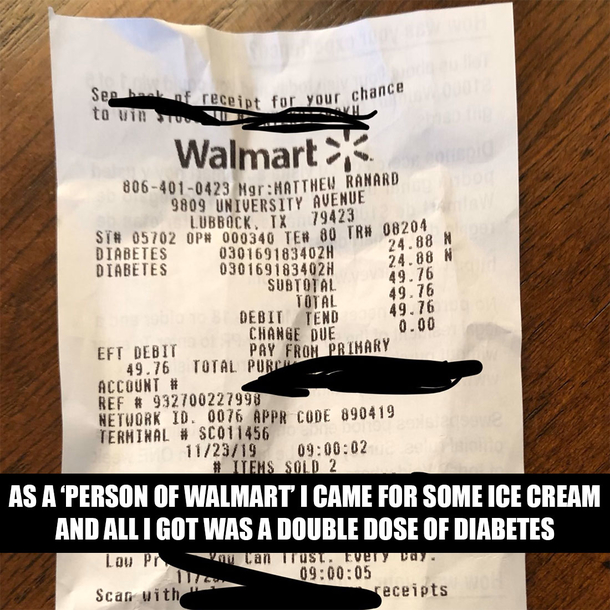